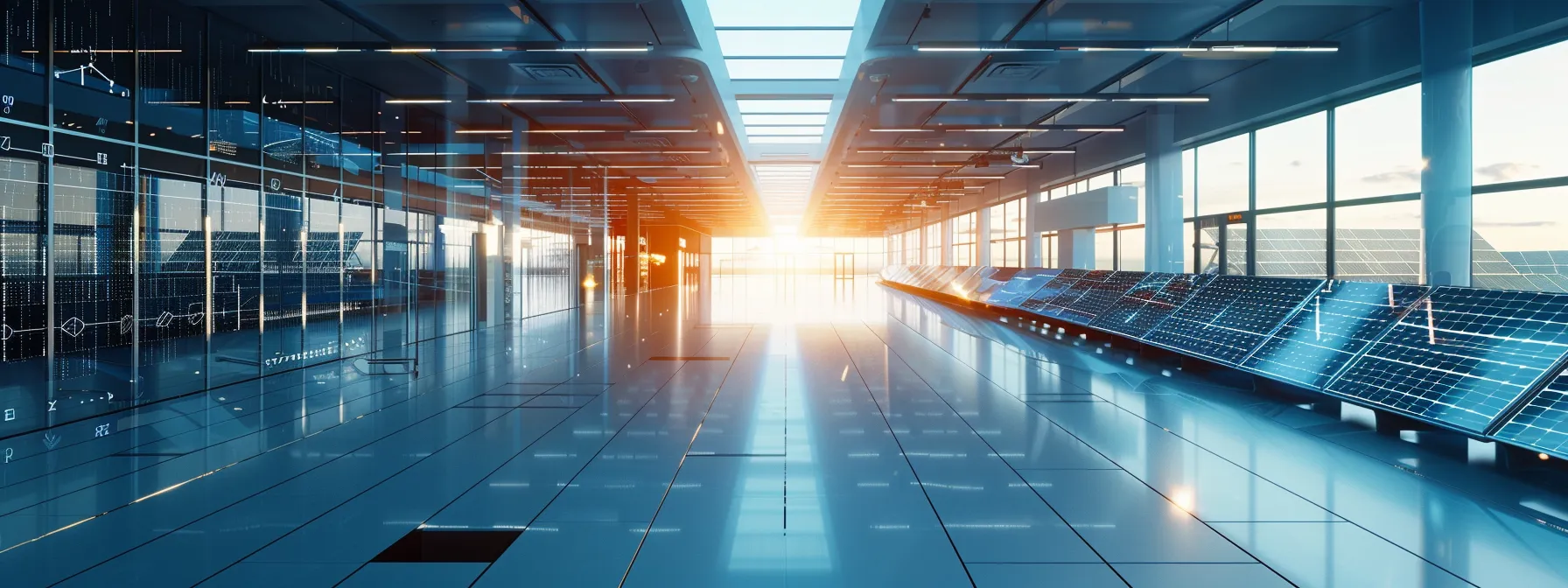
Are you looking to improve the reliability and efficiency of your solar panels? With the increasing adoption of AI, solar panel predictive maintenance is becoming more advanced and effective. This article will explore the foundational concepts of solar maintenance, the roles of artificial intelligence in monitoring panel conditions, and the methods that drive these improvements. By reading this content, you will understand how AI can optimize maintenance plans, reduce costs, and prevent costly downtimes, ultimately enhancing your solar energy investments. Let’s address the challenges of keeping your solar panels in top condition with innovative AI solutions.
Foundational Concepts of Solar Panel Predictive Maintenance
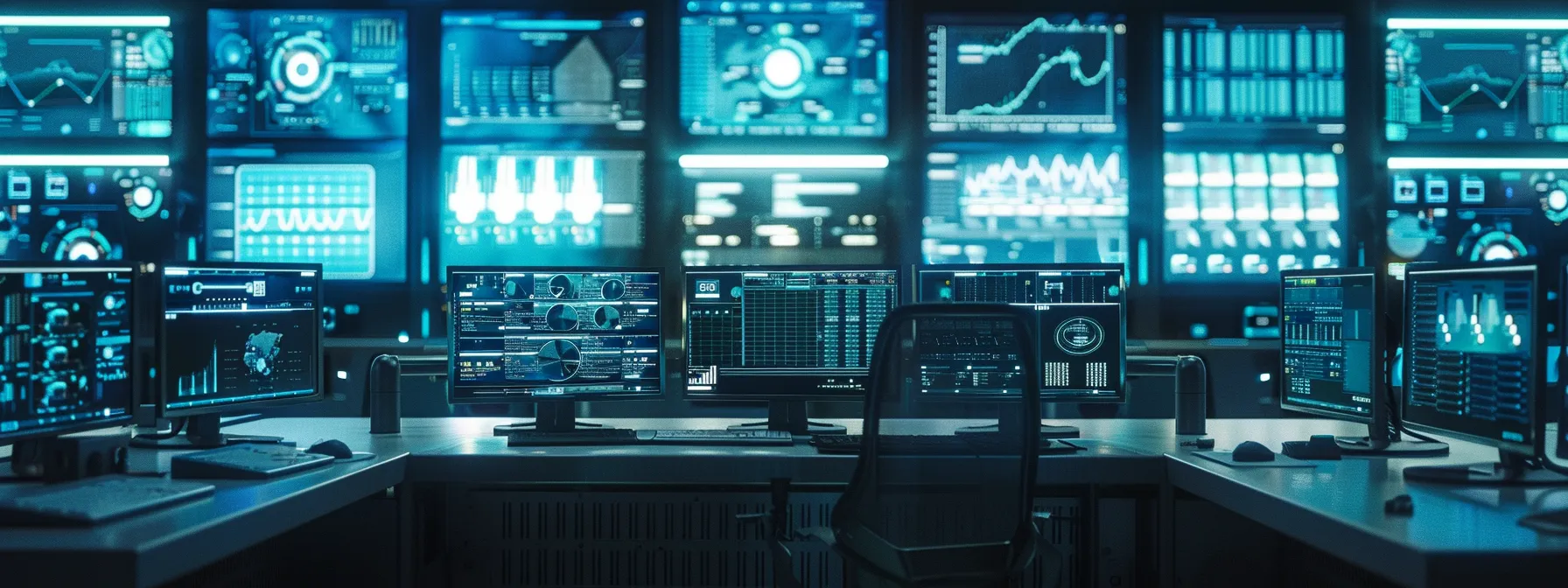
Proactive upkeep in solar installations involves anticipating and addressing potential issues before they arise. You’ll learn about how solar works, typical solar panel malfunctions and wear patterns, as well as the limitations of conventional servicing methods. Understanding the necessity for forward-thinking solar asset care will highlight how data analysis and intelligence can optimize performance, particularly in utility-scale applications, fostering a reliable energy curve through enhanced vibration monitoring.
Defining Proactive Upkeep for Solar Installations
Proactive upkeep for solar installations focuses on anticipating and resolving issues with equipment like solar inverters before they escalate into significant problems. Integrating a comprehensive company overview, we leverage advanced technologies such as the Internet of Things to monitor factors like sunlight exposure and wind conditions in real-time, leading to precise measurements and timely interventions. Understanding how solar works, this approach not only enhances the longevity of your solar assets but also ensures optimal energy production, addressing any potential inefficiencies in the system.
Typical Solar Panel Malfunctions and Wear Patterns
Common malfunctions in solar panels include performance degradation due to dirt accumulation, component failures in inverters, and issues arising from environmental factors like harsh weather. Monitoring these wear patterns through advanced systems such as SCADA and employing neural networks can significantly boost proactive maintenance efforts. By addressing these issues promptly, you enhance the return on investment for your solar assets, ensuring they contribute effectively to sustainable energy production over their lifespan. To understand how solar works, it’s essential to consider these factors. Additionally, exploring career opportunities in this field can lead to a rewarding and impactful career.
Shortcomings of Conventional Solar Panel Servicing Methods
Conventional solar panel servicing methods often rely on scheduled maintenance and visual inspections, which can lead to missed issues and inefficiencies. These outdated practices do not utilize advanced algorithms to analyze real-time data such as solar irradiance levels and performance metrics, making it challenging to proactively address problems that may impact sustainability. As a result, you may find that your solar assets underperform, which can affect your company’s energy output and overall return on investment.
The Requirement for Forward-Thinking Solar Asset Care
Adopting forward-thinking solar asset care is essential for maximizing the efficiency of your solar installations. By integrating predictive analytics and leveraging the industrial internet of things, you can harness data from various sensors to monitor system performance in real-time. This proactive approach enables you to pinpoint anomalies early, ensuring that your solar infrastructure consistently supports the electrical grid and meets energy demands effectively.
Understanding the essential principles of predictive maintenance lays the groundwork for smarter solutions. Now, let’s explore how artificial intelligence transforms the way we monitor solar panel health.
Artificial Intelligence Roles in Monitoring Solar Panel Condition
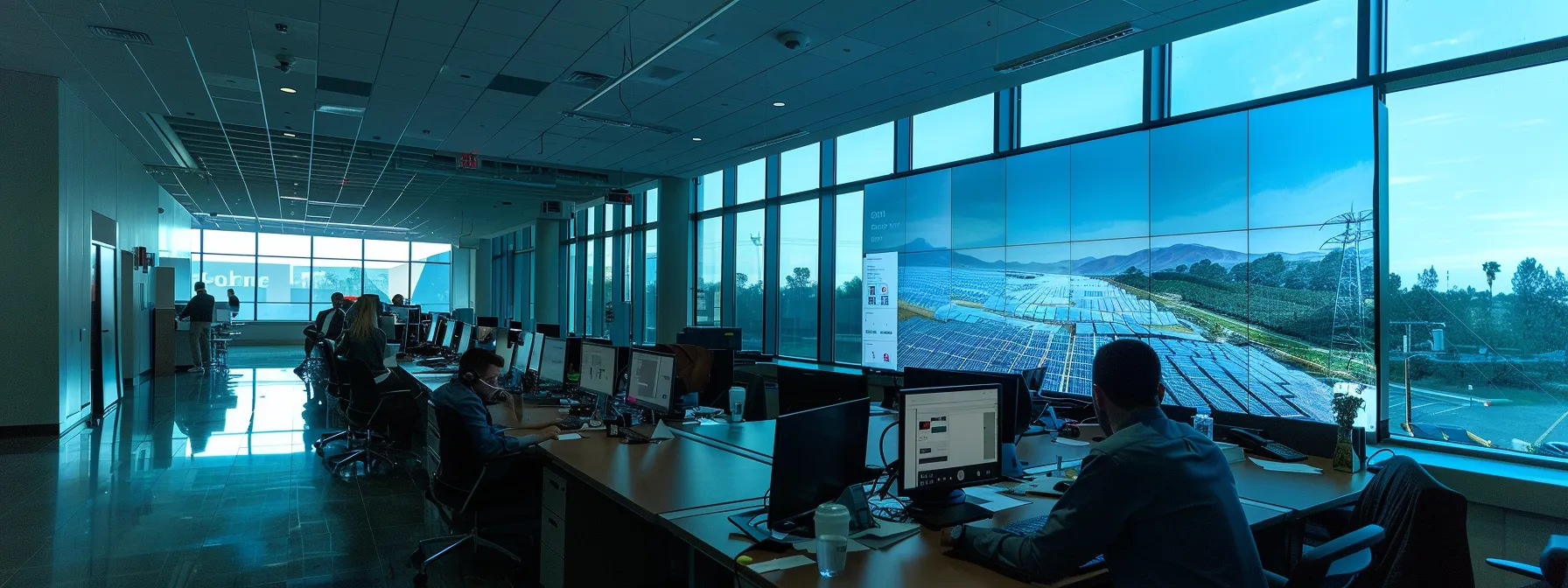
Employing machine learning allows for the identification of irregularities in solar power outputs by detecting patterns that suggest stress or malfunction. Utilizing deep learning for drone image interpretation offers insight into panel conditions, while AI enhances output prediction and triggers discrepancy warnings. Moreover, AI processes maintenance logs and technician notes, integrating diverse sensor inputs for comprehensive analysis, contributing to better-informed decisions.
This blend of advanced technologies aligns with ongoing research to optimize predictive maintenance, ensuring your solar assets operate at peak efficiency.
Employing Machine Learning for Identifying Irregularities
Employing machine learning for identifying irregularities in solar panel conditions is a game-changer in predictive maintenance. By utilizing advanced pattern recognition techniques, you can process vast amounts of data from inspections, detecting anomalies before they escalate into larger issues. This innovation allows you to maintain a detailed memory of system performance, facilitating timely adjustments that enhance operational efficiency and reliability.
- Leverage machine learning for real-time data analysis.
- Implement pattern recognition to identify potential issues early.
- Utilize insights from inspections to drive maintenance strategies.
- Enhance memory management for continuous performance monitoring.
- Adopt innovations in AI to streamline predictive maintenance processes.
Using Deep Learning for Drone Image Interpretation
Utilizing deep learning for drone image interpretation revolutionizes how you monitor solar panel conditions. By applying techniques rooted in supervised learning, you can analyze high-resolution images to detect issues like misalignment or panel damage before they escalate, ensuring the efficient operation of solar installations. This method not only enhances the reliability of energy production but also complements other renewable sources, such as wind power, in energy storage solutions, ensuring that your systems operate at optimal levels.
Applying AI for Output Prediction and Discrepancy Warnings
Applying AI for output prediction enhances the operational efficiency of solar installations by utilizing predictive modelling techniques to analyze historical performance data. Through feature engineering, you can create models that account for various environmental factors, enabling your infrastructure to respond proactively to performance discrepancies. This predictive capability not only extends the longevity of your solar assets but also ensures that they consistently meet energy demands, safeguarding your investment in renewable energy.
- Utilize predictive modelling for accurate output forecasting.
- Implement feature engineering to improve data analysis.
- Enhance operational efficiency with intelligent insights.
- Increase infrastructure longevity through proactive measures.
- Ensure consistent energy supply to meet demand.
How AI Processes Maintenance Logs and Technician Notes
AI plays a crucial role in processing maintenance logs and technician notes, transforming how you handle data acquisition for solar panel management. By utilizing logistic regression algorithms, AI can identify patterns that signal potential faults in equipment, allowing for proactive maintenance decisions based on historical data. This streamlining of asset management not only optimizes resource allocation but also enhances overall system reliability and performance through timely interventions.
- AI improves data acquisition from maintenance logs.
- Logistic regression identifies fault patterns efficiently.
- Streamlined asset management enhances resource allocation.
- Timely interventions support overall system performance.
Merging Diverse Sensor Inputs for AI Scrutiny
Merging diverse sensor inputs for AI scrutiny is essential for effective predictive maintenance of solar panels. By incorporating parameters from various sources, such as environmental sensors and performance metrics from associated wind turbine systems, you enable a comprehensive analysis that enhances risk management strategies. This multifaceted approach allows for accurate prediction of potential issues, significantly reducing maintenance expenses and ensuring that your solar assets operate at peak efficiency.
As we grasp how AI keeps a watchful eye on solar panels, it’s clear that its real power lies in predicting when things may go wrong. Let’s now explore the core methods that drive this predictive maintenance, bringing efficiency and savings to solar operations.
Core AI Methods Enabling Predictive Maintenance Gains
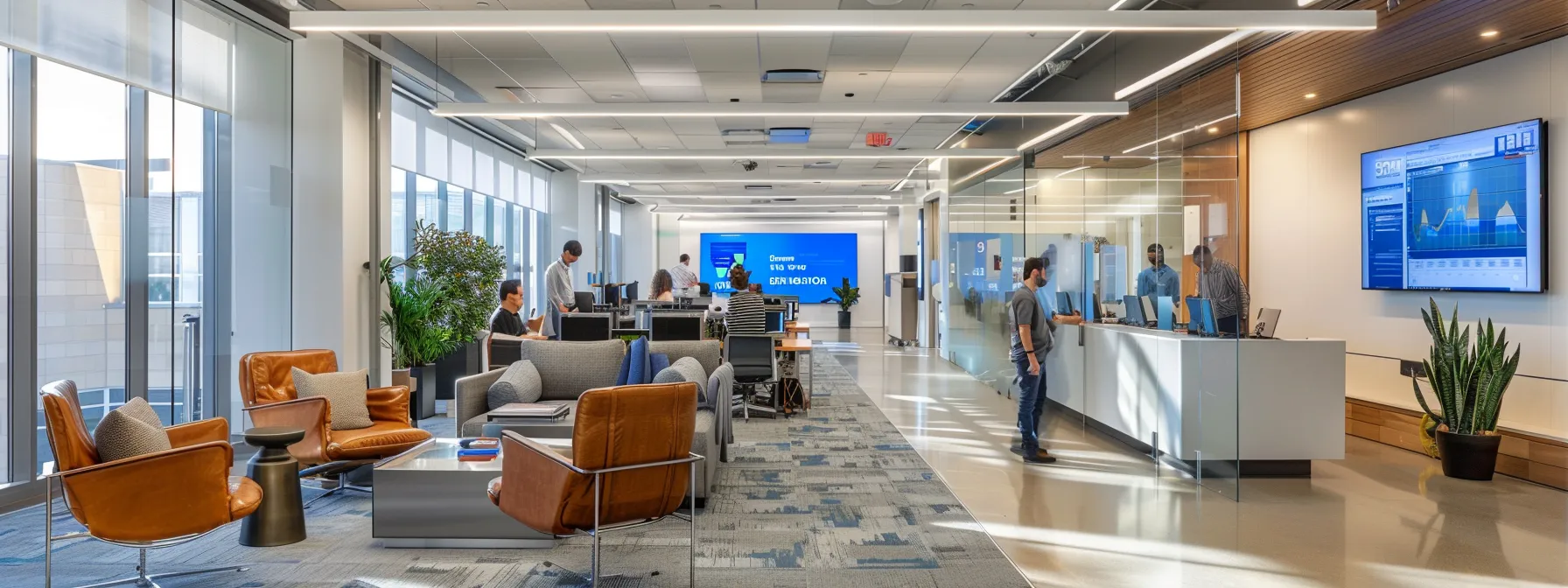
Key AI methods are transforming solar panel predictive maintenance by enhancing efficiency and condition monitoring. Supervised learning algorithms help you recognize known fault types, while unsupervised learning techniques identify unknown problems. Reinforcement learning applications optimize maintenance scheduling, and computer vision capabilities detect surface flaws. Furthermore, time-series forecasting aids in tracking output trends, ensuring robust data collection to inform finance solutions and support the creation of a digital twin for your solar assets.
Supervised Learning Algorithms for Recognizing Known Fault Types
Supervised learning algorithms play a vital role in recognizing known fault types within photovoltaic systems, enabling you to enhance the reliability and longevity of your solar energy installations. By analyzing historical data and warranty claims, these algorithms identify patterns associated with specific cell malfunctions, allowing for early detection of issues before they escalate. This proactive approach not only streamlines the supply chain for replacement parts but also boosts overall system performance, ensuring your solar assets consistently deliver optimal energy output.
Unsupervised Learning Techniques for Finding Unknown Problems
Unsupervised learning techniques play a crucial role in a predictive maintenance system for photovoltaics by identifying unknown problems that could pose risks to your solar assets. These methods analyze behavioral patterns and anomalies in system performance without needing pre-labeled training data. By monitoring real-time data, you can uncover hidden issues that traditional maintenance approaches might overlook, ultimately enhancing the reliability and efficiency of your solar installations.
Reinforcement Learning Applications in Maintenance Scheduling
Reinforcement learning applications in maintenance scheduling are pivotal for optimizing your solar panel systems. By using algorithms that adapt based on outcomes, you can efficiently manage server resources to predict maintenance needs tied to factors like irradiance fluctuations and system performance. This approach minimizes the potential for failures, ensuring your renewable energy assets operate effectively and consistently meet energy demands.
- Utilize reinforcement learning to adapt maintenance schedules based on real-time data.
- Monitor irradiance levels to predict operational needs and reduce failures.
- Leverage server analytics to enhance resource management in your solar assets.
- Implement insights from maintenance data to improve overall system performance.
Computer Vision Capabilities for Spotting Surface Flaws
Computer vision capabilities significantly enhance anomaly detection in solar panels by enabling precise identification of surface flaws. Through sophisticated image analysis, this technology allows you to monitor solar installations in real-time, detecting issues such as cracks, dirt accumulation, or misalignment that can impact energy production. By integrating this data into a centralized database, you can ensure a comprehensive overview of your assets, ultimately optimizing the performance of your solar operations as outlined on the solarai homepage.
- Utilize computer vision for real-time monitoring of solar panel conditions.
- Implement advanced image analysis for effective anomaly detection.
- Create a centralized database to optimize solar asset management.
- Ensure consistent energy production through proactive maintenance strategies.
Time-Series Forecasting for Tracking Output Trends
Time-series forecasting is a vital tool in tracking output trends for renewable energy systems, particularly in solar installations. This method enables you to analyze historical performance data and identify patterns related to shading and other environmental factors that may impact energy production. By leveraging machine learning algorithms, you enhance the accuracy of your forecasts, ensuring that your solar assets maintain optimal performance and effectively meet energy demands.
- Utilize time-series forecasting to analyze historical performance data.
- Identify patterns relating to shading and other environmental factors.
- Leverage machine learning algorithms to improve forecast accuracy.
- Ensure optimal performance of solar assets in meeting energy demands.
With core AI methods in place, the path to effective solar maintenance comes clear. Now, let’s examine the tangible gains that follow from well-informed, AI-guided plans.
Measurable Benefits of AI-Guided Solar Maintenance Plans
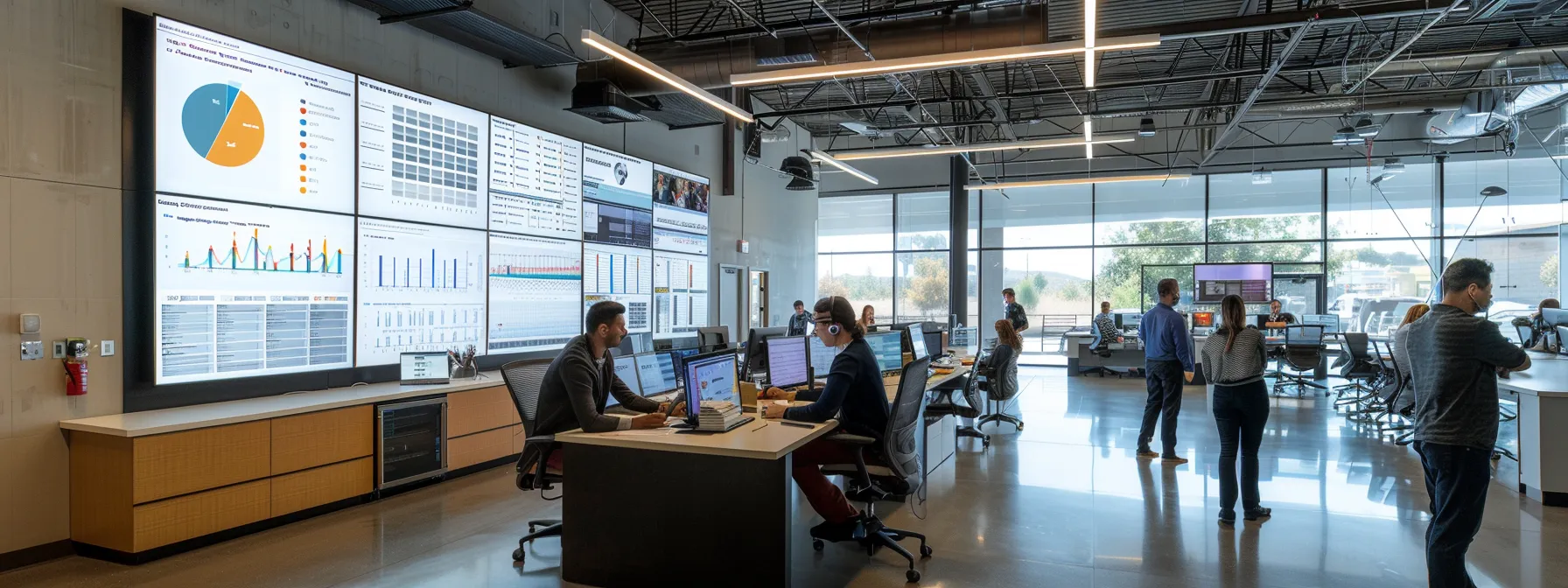
Implementing AI-guided solar maintenance plans allows you to achieve greater energy output by minimizing interruptions. These intelligent systems reduce operational and repair costs while prolonging the lifespan of solar power equipment. You’ll also experience improved safety conditions for service crews and streamlined deployment of maintenance resources. Each of these advantages directly enhances the overall efficiency and reliability of your solar operations.
Attaining Greater Energy Output via Minimized Interruption
Minimizing interruptions in solar panel operation directly correlates to achieving greater energy output, and AI-driven maintenance plans are key to this strategy. By leveraging predictive analytics, you can anticipate potential failures and perform maintenance before issues escalate, ensuring that your solar systems operate continuously at peak performance. This proactive approach reduces downtime, allowing your installations to generate electricity efficiently and consistently, which translates into enhanced returns on your investment in renewable energy.
Reducing Costs Associated With Operations and Repairs
Utilizing AI-guided maintenance strategies significantly reduces operational and repair costs in solar installations. By predicting potential equipment failures before they occur, AI enables you to perform maintenance only when necessary, avoiding unnecessary inspections and repairs. This proactive approach minimizes labor expenses and extends the lifespan of solar assets, ultimately leading to a more cost-effective and efficient energy production model.
Prolonging the Service Duration of Solar Power Equipment
Prolonging the service duration of solar power equipment is significantly enhanced through the implementation of AI-guided maintenance plans. By utilizing predictive analytics, you can anticipate equipment failures before they occur, allowing for timely interventions that prevent more serious issues. This proactive strategy not only extends the lifespan of your solar assets but also ensures they operate efficiently over time, maximizing your return on investment in renewable energy.
- Anticipate equipment failures through predictive analytics.
- Enable timely interventions to prevent serious issues.
- Extend the lifespan of solar assets effectively.
- Ensure efficient operation over time.
- Maximize return on investment in renewable energy.
Bettering Safety Conditions for Service Crews
Bettering safety conditions for service crews is a critical benefit of AI-guided solar maintenance plans. By utilizing predictive analytics, you can forecast equipment failures and avoid hazardous situations before they occur, ensuring that technicians have a safer work environment. Furthermore, real-time monitoring of solar panel conditions reduces the need for unnecessary inspections, allowing service crews to focus on essential tasks while minimizing their exposure to potential dangers associated with maintenance work.
Streamlining How Maintenance Resources Are Deployed
Streamlining how maintenance resources are deployed is essential for maximizing the efficiency of your solar operations. By employing AI-driven analytics, you can allocate maintenance teams strategically, focusing on areas that require urgent attention based on real-time performance data. This targeted approach reduces unnecessary travel and downtime, allowing your technicians to concentrate on high-priority tasks while optimizing resource utilization effectively.
- Anticipate issues early through predictive analytics.
- Allocate maintenance crews based on immediate needs.
- Reduce travel and downtime with real-time data integration.
- Enhance focus on high-priority tasks for improved performance.
- Maximize resource utilization for efficient solar maintenance.
With clear benefits in hand, you now seek the next steps. Implementing AI for solar panel care can change the game entirely.
Putting AI Systems Into Practice for Solar Panel Care
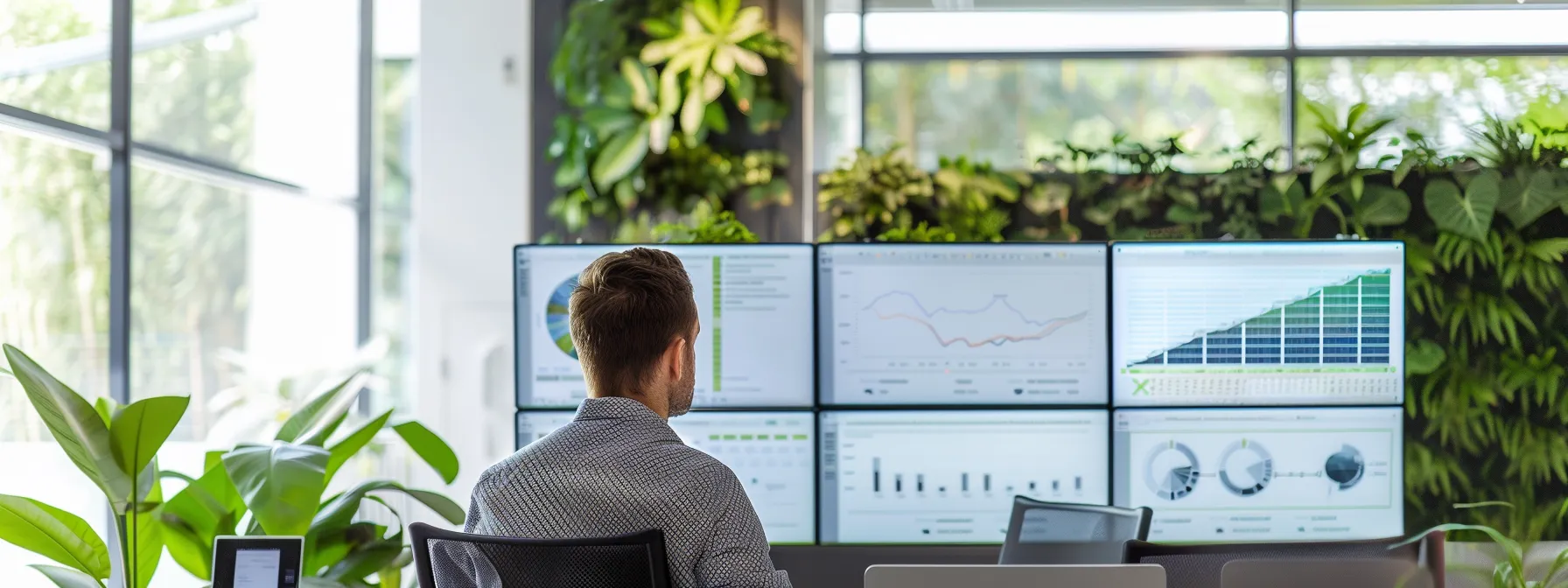
Choosing suitable sensors and data gathering tools is vital for effective AI-driven predictive maintenance in solar panel care. You’ll also consider selecting the right AI framework or software solution to process the collected data accurately. Steps for data cleansing and AI model development will ensure high-quality insights, while integrating AI findings into standard maintenance procedures enhances operational efficiency. A real-world example will demonstrate the successful adoption of AI in optimizing solar maintenance practices.
Choosing Suitable Sensors and Data Gathering Tools
Choosing suitable sensors and data gathering tools is crucial for enhancing solar panel predictive maintenance through AI integration. By utilizing high-quality environmental sensors, you can monitor factors such as temperature, irradiance, and atmospheric conditions in real-time, providing critical data for predictive analytics. This comprehensive understanding of your solar assets enables you to address potential issues proactively, ensuring optimal performance and maximizing energy output.
Selecting an Appropriate AI Framework or Software Solution
Selecting an appropriate AI framework or software solution for solar panel predictive maintenance is essential to optimize performance effectively. You should consider platforms that offer robust data processing capabilities and integrate seamlessly with existing sensor systems. Look for solutions that provide actionable insights through predictive analytics, enabling you to address potential issues proactively and enhance the longevity of your solar assets.
Steps for Data Cleansing and AI Model Development
To effectively implement AI in solar panel predictive maintenance, you must start with meticulous data cleansing and AI model development steps. Begin by gathering data from various sensors and ensuring that it is free from inaccuracies and irrelevant information. This process may involve removing duplicates, handling missing values, and normalizing data to create a reliable dataset for training AI models, ensuring optimal predictive performance.
- Gather data from various sensors.
- Remove inaccuracies and irrelevant information.
- Handle missing values and normalize data.
- Prepare a reliable dataset for training AI models.
- Ensure optimal predictive performance through thorough cleansing.
Weaving AI Findings Into Standard Maintenance Procedures
Integrating AI findings into standard maintenance procedures is crucial for optimizing solar panel performance. By utilizing insights derived from predictive analytics, you can enhance decision-making processes, ensuring that maintenance strategies are based on real-time data rather than outdated practices. This proactive approach allows you to streamline operations, reduce downtime, and maximize the energy output of your solar installations, ultimately leading to a more sustainable and profitable energy model.
Real-World Example Showcasing Effective AI Adoption
One notable example of effective AI adoption in solar panel predictive maintenance comes from a large utility-scale solar farm that implemented machine learning algorithms to monitor system performance. By analyzing real-time data from various sensors, the facility was able to identify equipment anomalies early, which led to timely interventions and decreased downtime significantly. This proactive approach not only optimized energy output but also reduced operational costs, showcasing how AI can transform solar maintenance practices and enhance overall asset efficiency.
AI is proving its worth in the daily care of solar panels. Yet, there are new developments on the horizon, ready to reshape maintenance in solar facilities.
Emerging Developments for AI in Solar Facility Upkeep
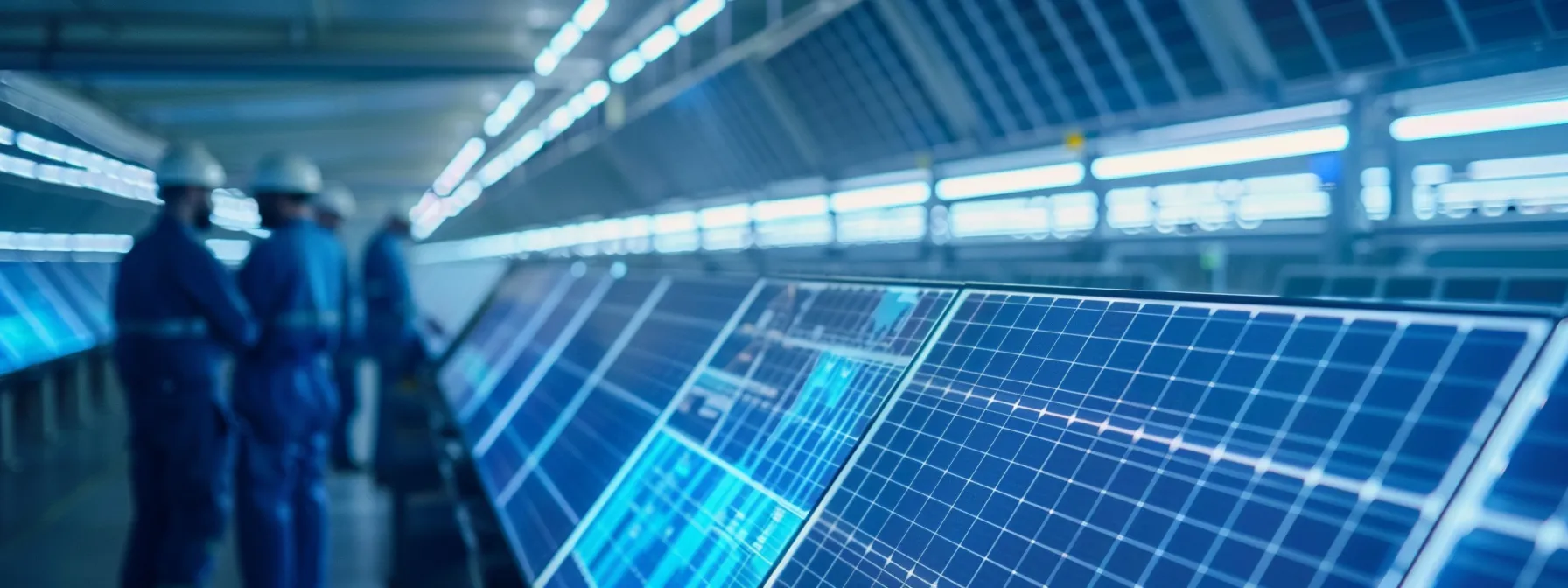
Edge computing AI significantly contributes to instantaneous field analysis, allowing for real-time decision-making. Advances in automated drone surveys utilize AI to enhance inspection accuracy, while pairing AI analysis with digital replicas of solar plants offers deeper insights into performance. Furthermore, AI can forecast specific part breakdowns, addressing the challenges of data protection and algorithmic openness effectively.
These developments represent a convergence of innovative technologies that optimize solar facility upkeep, ensuring your solar assets operate at peak efficiency and reliability.
Contribution of Edge Computing AI to Instantaneous Field Analysis
Edge computing AI revolutionizes solar panel predictive maintenance by enabling instantaneous field analysis of data collected from sensors. By processing information at the source, you can achieve real-time monitoring of equipment conditions and make quick, informed decisions to address potential issues before they escalate. This approach minimizes downtime and enhances the efficiency of your solar operations, ensuring that each panel performs optimally and contributes effectively to your energy goals:
- Real-time data processing at the source improves reaction times.
- Quick decision-making minimizes potential downtimes.
- Enhanced monitoring leads to optimized panel performance.
Progress in Automated Drone Surveys Guided by AI
Progress in automated drone surveys guided by AI is transforming how you monitor solar facilities. By utilizing drones equipped with advanced imaging technology, you can conduct thorough inspections of solar panels with unprecedented speed and accuracy. This method not only detects issues such as misalignment or damage early but also facilitates comprehensive data collection, allowing for proactive maintenance that enhances the overall efficiency and reliability of your solar installations.
Pairing AI Analysis With Digital Replicas of Solar Plants
Pairing AI analysis with digital replicas of solar plants enhances predictive maintenance by providing real-time insights into equipment conditions. These digital twins allow you to simulate various operational scenarios and assess potential issues before they arise. By integrating AI algorithms with these replicas, you can accurately forecast equipment behavior, enabling proactive measures that safeguard your solar investments and ensure optimal energy production.
Possibilities for AI Forecasting Specific Part Breakdowns
AI-driven forecasting of specific part breakdowns allows you to enhance the reliability of your solar installations significantly. By utilizing machine learning algorithms, you can analyze historical performance data and identify patterns that predict when components, such as inverters or batteries, are likely to fail. This proactive approach not only reduces unexpected downtimes but also enables you to allocate maintenance resources more effectively, ensuring the longevity and efficiency of your solar assets:
- Identify patterns in historical performance data.
- Predict breakdowns of key components like inverters or batteries.
- Enhance resource allocation for maintenance efforts.
- Reduce unexpected downtimes and improve system reliability.
Meeting Challenges of Data Protection and Algorithmic Openness
Addressing challenges related to data protection and algorithmic openness in AI for solar panel predictive maintenance is crucial for ensuring trust and reliability in your systems. As you implement advanced AI technologies, it will be essential to establish robust data governance frameworks that safeguard sensitive information while promoting transparency in algorithm operations. By adopting best practices such as encryption and regular audits, you can enhance data security and optimize algorithm performance without compromising your intellectual property or operational integrity.
- Establish robust data governance frameworks to safeguard sensitive information.
- Utilize encryption and regular audits to enhance data security.
- Promote transparency in algorithm operations to build trust with stakeholders.
- Balance data protection with the need for openness in AI processes.
- Implement best practices to improve both security and operational integrity.
Conclusion
AI significantly enhances predictive maintenance for solar panels by proactively identifying potential issues, optimizing efficiency, and reducing operational costs. Through advanced data analysis and machine learning, operators can anticipate equipment failures and facilitate timely interventions, ensuring that solar assets consistently perform at their best. This approach not only extends the lifespan of equipment but also maximizes energy output, contributing to a sustainable energy future. Embracing AI-driven strategies empowers professionals in the solar industry to elevate their operations and ensure reliable renewable energy production.